Project 5: Development of online coupled data assimilation capability for R-CESM and CESM
Project Leaders
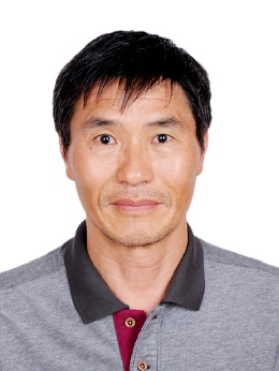
Zhang (QNLM)
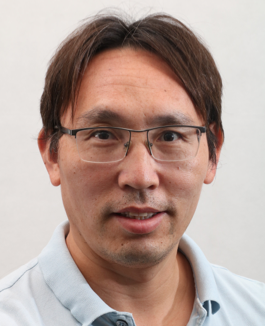
Liu (TAMU)
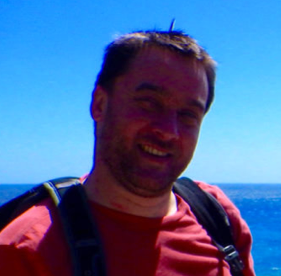
Castruccio (NCAR)
Project Objective
A key requirement for skillful climate prediction is accurate initial conditions that can be achieved by data assimilation (DA) procedures. Coupled data assimilation (CDA) approach is developed to address imbalance among model states in different components. In this approach, DA is performed within a coupled model framework (Figure). There are rapid developments in CDA research in recent years, following the pioneering CDA efforts at the US National Centers for Environmental Prediction (NCEP) Climate Forecast System (CFS) (Saha et al. 2006, 2010) and at the Geophysical Fluid Dynamics Laboratory (GFDL) (Zhang et al. 2007). CDA has reached a stage that shows a great potential for developing the ability of seamless weather and climate predictions (Brunet et al. 2015).
The objective of this research project is to test a working hypothesis that online CDA provides the most effective and efficient means of initializing high-resolution Earth System models and can improve model forecast skills on subseasonal-to-decadal time scales.
Research Plan
To test this hypothesis, we will first develop an online ensemble Kalman filter (EnKF) based DA capability for the ocean component model, ROMS, within the framework of R-CESM configured for the Gulf of Mexico and performed a partial CDA simulation, where DA is only applied to the ocean component while running fully coupled R-CESM at eddy-resolving resolution in the Gulf of Mexico. Next, we will develop an online DA capability for the atmospheric component model, WRF, within the R-CESM framework and perform full CDA simulations for the Gulf of Mexico.
In parallel with the effort in developing the online CDA capability for R-CESM, we will develop and implement a new online CDA scheme to the low-resolution version of CESM. The new scheme is based on the Multi-Timescale EnOI-like High-Efficiency Approximate Filter (MSHea-EnKF) recently developed by Zhang et al. (2019). This scheme combines the optimal interpolation approach with the EnKF approach to allow assimilating multi-timescale observations into coupled Earth System in a more efficient manner.
Project time table
Year 1
- Complete the development and test of DA-enabled ocean component within R-CESM.
- Conduct partial CDA simulations using R-CESM in the Gulf of Mexico.
- Begin implementing MSHea-EnKF to low-resolution CESM.
Year 2-3
- Complete the development and implementation of DA to the atmospheric component of R-CESM.
- Conduct full CDA simulations in the Gulf of Mexico and compare the results to those of partial CDA simulations.
- Complete the implementation of MSHea-EnKF to low-resolution CESM.
Year 4-5
- Conduct regional hindcast experiments in the Gulf of Mexico with and without CDA.
- Conduct global CDA simulations using low-resolution CESM.
- Publish the results of CDA-enabled R-CESM and the results of CDA-enabled low-resolution CESM simulations.
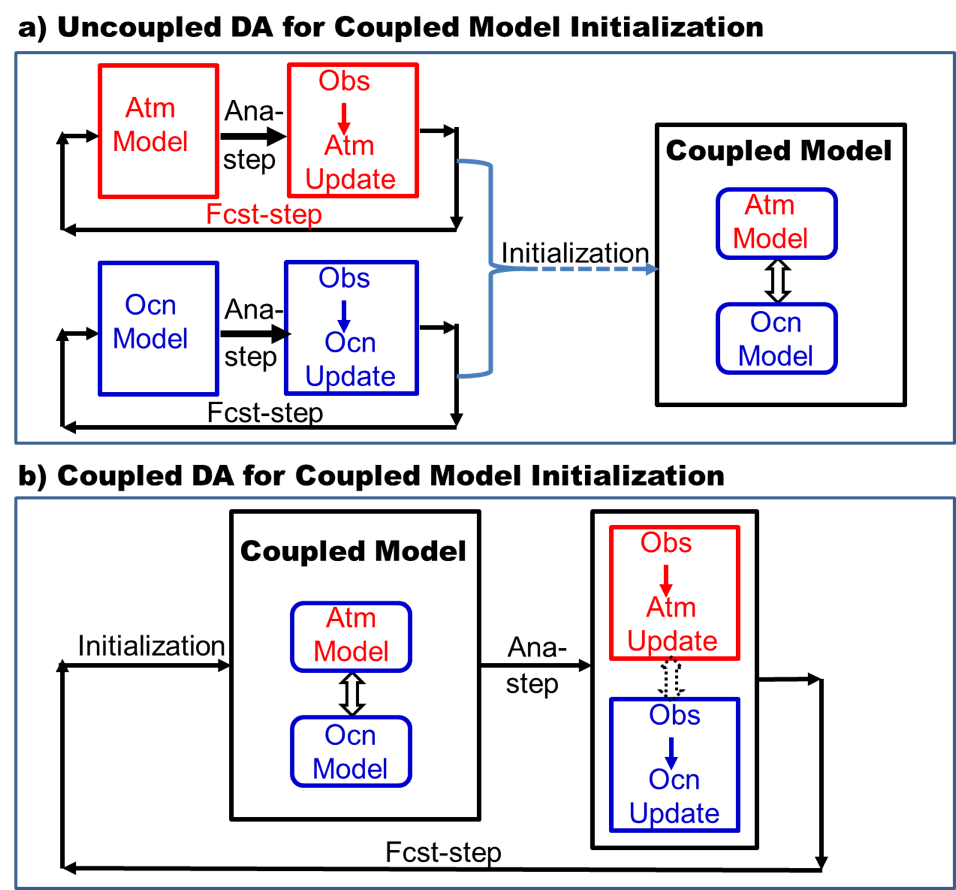
Schematic illustration of a) uncoupled data assimilation and b) coupled data assimilation. While uncoupled data assimilation (panel a) uses the forecasts of uncoupled atmosphere and ocean models as the first-guesses in the atmospheric and oceanic analysis processes (marked by the red and blue arrows), the first-guesses of coupled data assimilation analysis processes (panel b) are the forecasts of the whole coupled model. The initialization of climate prediction is a natural consequence of coupled data assimilation while uncoupled data assimilation takes the combination of separate atmosphere data assimilation and ocean data assimilation results to initialize the coupled model for climate prediction. The dashed double-arrow between the atmospheric and oceanic analysis updates implies that within the coupled data assimilation framework, the atmosphere (ocean) observations can directly impact on ocean (atmosphere) model states through cross-covariance between the atmosphere and ocean states, i.e. implementing strongly coupled data assimilation. Otherwise if only coupled model forecasts are used as the first-guess in the analysis update (without direct observational impact cross the atmosphere and ocean), then weakly coupled data assimilation is implemented.
Progress and Current Status
The QNLM team has developed a new high-efficiency data assimilation algorithm that is better suited for high-resolution coupled earth system models. The new scheme is named multi-time scale, high-efficiency approximate EnKF (MSHea-EnKF) and takes into consideration of multi-time scale nature of the background error statistics, consisting of stationary, slow-varying, and fast-varying parts. It is a combination of multi-time scale filters implemented by regressions based on data sampled from the time series of a single model solution rather than an ensemble of runs. This allows computational efficiency, making it more applicable to high-resolution models. The work has been published in Journal of Advances in Modeling Earth System (JAMES) (Yu et al. 2018).
Research & Projects
ResearchModeling ToolsProject 1: High-resolution CESM present and future climate simulationsProject 2: High-resolution ensemble CESM decadal climate predictionProject 3: High-resolution ocean model improvement and development Project 4: Development of R-CESM and online nesting capability for CESMProject 5: Development of online coupled data assimilation capability for R-CESM and CESM